In a previous post, I expressed my frustration with the term “hallucination” when used to describe the inaccuracies of large language models. This term creates a misconception that AI tools have agency, hiding the complexities of marketing hype and other factors — such as plain old nonsense or in other words bovine excrement bullshit!!
It’s tempting and easy to anthropomorphise technology, talking about it as if it were a person. But we all fall for it. Researchers and reporters should steer clear of clickbaity headlines that exaggerate AI’s capabilities. But I don’t see this trend slowing down anytime soon.
Consider a recent (May 10, 2024) headline (The Guardian) like “Is AI lying to me? Scientists warn of growing capacity for deception.” No, AI is not and cannot lie to you! This article is full of anthropomorphic bait that is cringeworthy, even though the underlying problems (AI generated misinformation, misuse of AI, lack of transparency) are real. More accurate headlines might be: “Is the AI providing incorrect information?" or “Is the AI generating inaccurate responses?” These alternatives are straightforward and avoid the pitfalls of anthropomorphism.
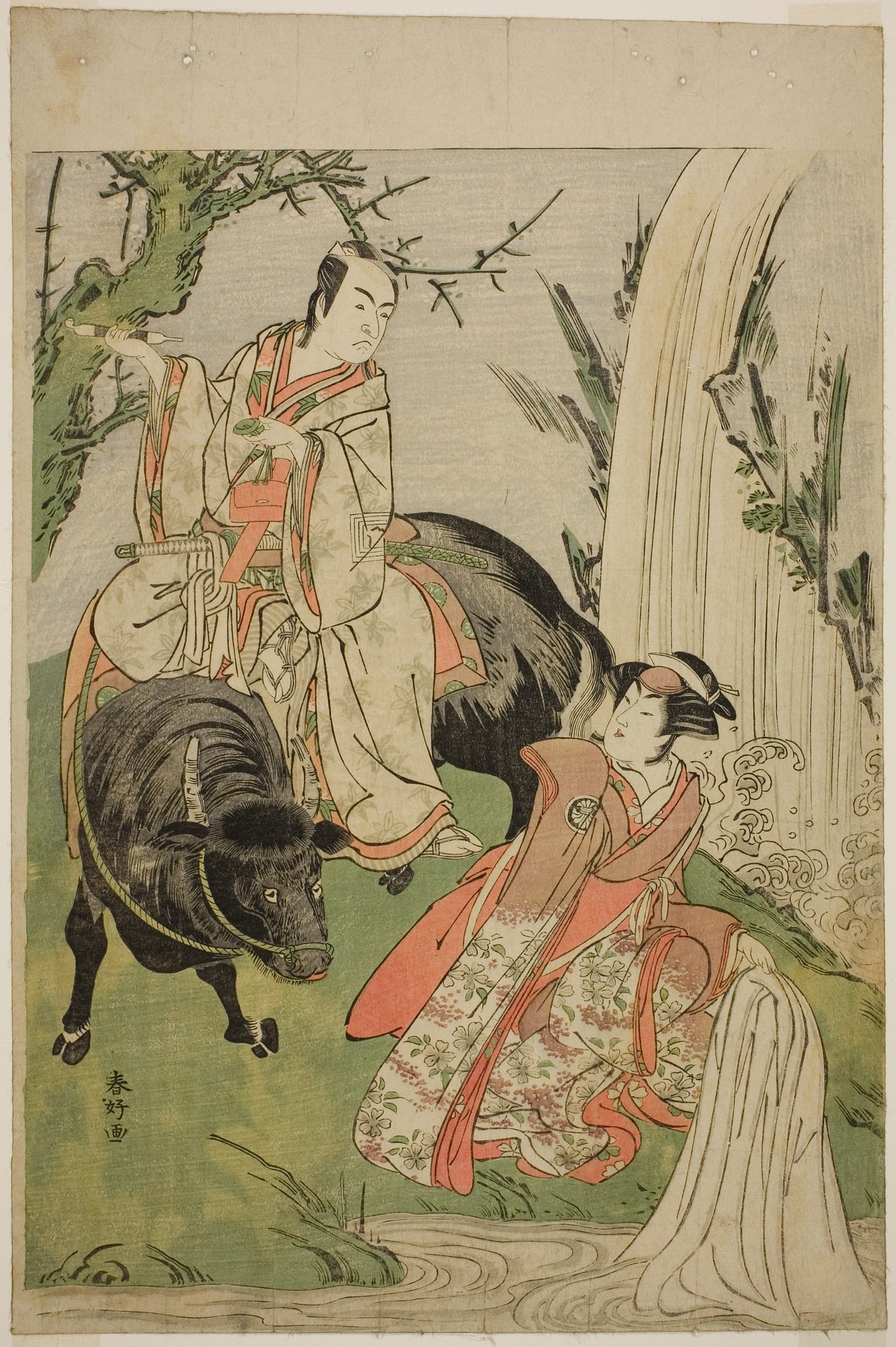
There’s also now a growing emphasis on AI safety, which, while commendable, can often fall into the trap of anthropomorphism. Our understanding of reasoning and intelligence, especially in the context of AI, remains limited. This can lead to computer scientists, who may not have extensive training in ethics, philosophy, or social sciences, defining AI safety based on human concepts like honesty, helpfulness, and harmlessness.
Professor Anthony Cohn, from the University of Leeds and the Alan Turing Institute, who was quoted in the above Guardian article, highlighted the challenge of defining desirable (??!!! who decides this!!) and undesirable (wtf??) behaviours for AI systems:
“Desirable attributes for an AI system (the “three Hs”) are often noted as being honesty, helpfulness, and harmlessness, but as has already been remarked upon in the literature, these qualities can be in opposition to each other: being honest might cause harm to someone’s feelings, or being helpful in responding to a question about how to build a bomb could cause harm,” he said. “So, deceit can sometimes be a desirable property of an AI system. The authors call for more research into how to control the truthfulness which, though challenging, would be a step towards limiting their potentially harmful effects.”
No, deceit cannot be a property. Because it is difficult to design AI models that cannot be misused. Even if AI could not generate false information, it could still be used to spread disinformation by creating misleading contexts around truthful information. Just like computers, AI models are general-purpose tools and can be applied for both good and bad purposes. Here’s a relevant quote from a recent article in the excellent newsletter AI Snake Oil:
We see the same pattern over and over. There has been alarm about LLMs being able to give bioterrorists information on how to create pathogens. But that information is readily available on the internet. The hard parts for would-be bioterrorists are all of the other steps involved: obtaining raw materials, culturing cells in the lab without killing them or infecting oneself, and disseminating the bioweapon to cause harm. AI could potentially aid that work, as it is a general-purpose tool and has some usefulness for almost all knowledge work. Again, this illustrates the limits of attempting to build safety into models: most of the questions the user would ask in this process relate to synthetic biology in general and not bioweapons in particular. To be sure that a model couldn’t assist bioterrorists, it would have to refuse to assist with any sort of bioengineering.
Or consider the use of LLMs to generate disinformation. Even in the unlikely event that a model could be aligned so that it refuses all requests to generate false information, research has found that true-but-misleading information is far more impactful than false information on social media; 50x more in the case of increasing vaccine hesitancy. So even a hypothetical safe model could be used to aid disinformation efforts: the adversary would use it to generate factual information (e.g. accurately summarizing news stories), with the misleading context added in separately.
In short, trying to make an AI model that can’t be misused is like trying to make a computer that can’t be used for bad things.
Again, the use of AI in every realm of decision making causes us to bring these issues in the forefront. But remember AI is neither being honest or dishonest. Try to understand the underlying structure and context because using the term hallucination!
While I am getting frustrated reading the same old tropes from the hype machine, I found a compelling historical explanation of the term hallucination’ in AI, of all places, at the LA Review of Books. Joshua Pearson’s (who teaches courses in science fiction, media, and cultural studies at California State University, Los Angeles) article traces the history of this term and criticises its use. He argues that calling AI outputs hallucinations evokes misleading associations with human cognition, mystifying AI’s true capabilities and limitations.
According to Pearson’s research, the term hallucination in AI has roots in early text and image processing research. In 1982, John Irving Tait used hallucination to describe a text-parsing issue where the system saw a text that fit its expectations rather than the actual input. In 1985, Eric Mjolsness used the term for a system that improved fingerprint images by creating clean patterns from random input. Simon Baker and Takeo Kanade’s 1999 paper, “Hallucinating Faces,” described generating high-resolution images from low-pixel inputs, with additional pixels being “hallucinated” by the algorithm. But it was less in anthropomorphising form then. From Joshua Pearson:
Like many AI skeptics today, Tait locates the problem not in the faulty results but in the way the system is designed to make those outputs indistinguishable from accurate ones. While the text-processing side of the genealogy of “hallucination” does provide useful framing for today’s AI boosters, there is also more skepticism and concern for the end user than modern commentators might expect.
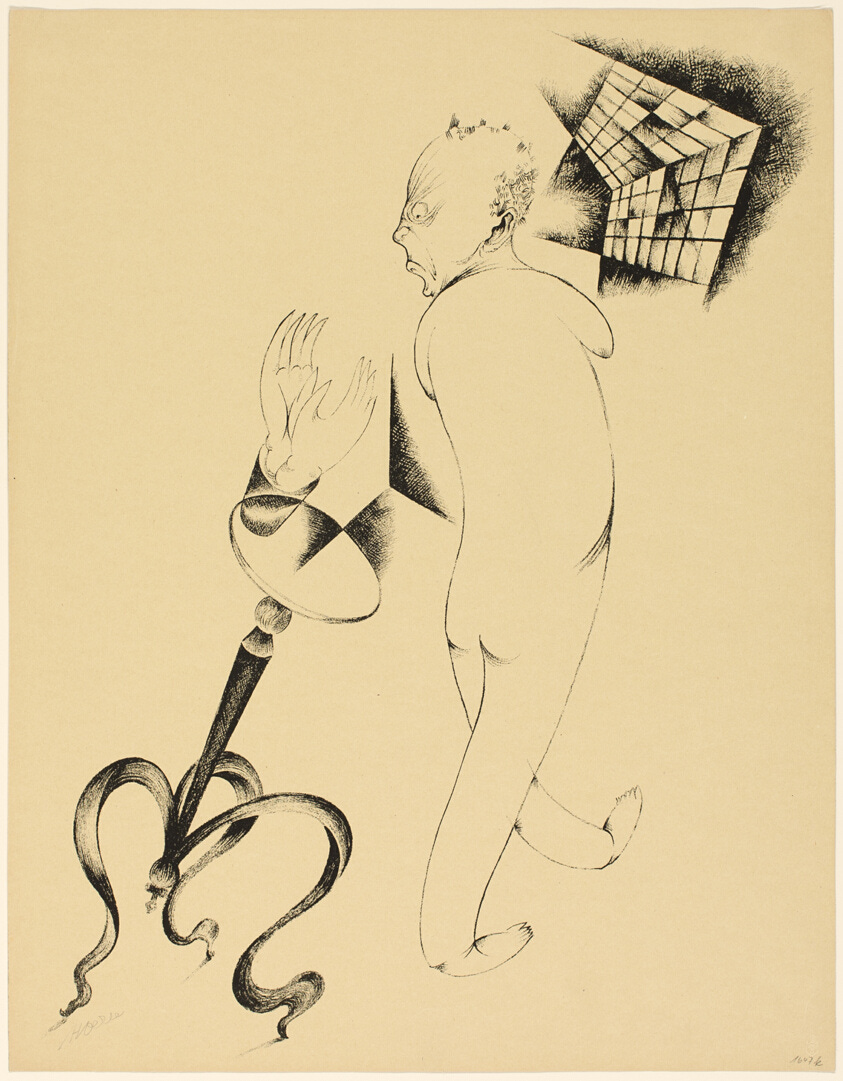
The bottomline is this …in the past, hallucination in AI meant creating something seemingly real, not necessarily true. Today, in AI, it describes unusual mistakes by otherwise good systems. This is where the problem lies.
Pearson and others argue that hallucination serves to obscure the real nature of AI outputs. Understanding the historical context of hallucination in AI reveals its true nature: AI outputs aim to seem right, irrespective of truth. By misrepresenting these outputs as rare errors, the term hallucination obscures the inherent nature of AI systems. This serves the interests of those who benefit from the hype and mystification surrounding AI technology.
Pearson refers to these earlier research and reframes hallucination as bullshit which is I think is more accurate and reflects the whole industry trend now. The hype machine found a way to frame an acknowledgment that AI output isn’t totally trustworthy while emphasising the idea that its output is still a generally accurate reporting of reality. That is the crux of the problem. This is clear when we use the term Bullshit. Researcher like Carl T. Bergstrom & C. Brandon Ogbunu wrote extensively on this:
A large language model, however, does not experience sense impressions, nor does it have beliefs in the conventional sense. Language that suggests otherwise serves only to encourage the sort of misconceptions that have pervaded popular culture for generations: that instantiations of artificial intelligence work much like our brains do….
“Bullshitting” may not have the same air of scientific formality as “hallucination” or “confabulation,” but it is closer to the truth of the matter. And the language we use for AI is consequential. It shapes the way we use and interact with the technology, and how we perceive its errors.
Whether you call it hallucination or bullshit, the problem is as old as bovine excrement. Technology and its usage are context-dependent, and there’s power and politics involved. The people who create these tools are providing us with convincing semblances rather than accurate representations. The challenge lies in how we contextualise errors generated by AI. Referring to AI as “hallucinating” implies that it sometimes gets things right, suggesting it’s not a default feature. However, as noted earlier, concepts like hallucination and honesty cannot be modelled as inherent AI properties. Context is crucial to understanding where and how AI is applied. I highly recommend the LA Review of Books article on hallucination for further insights. By understanding its historical context, the term "hallucination" reveals the inherent nature of AI outputs: they aim to seem right, irrespective of the truth.
Insightful read! Thanks a bunch 🙂