In the vast realm of our random universe, we are often entangled in a tapestry of uncertainty. It's a place where Schrödinger's cat, forever suspended between life and death, turns into a post-punk goth emo singer. Meanwhile we try to understand when the app says 20% chances of rain — should we pack our umbrellas or not. While we possess the capacity to make decisions based on factual evidence and keen observations, a realm of probabilistic outcomes exists beyond the reach of our control. At the same time we yearn for predictable and certain outcomes, seeking solace in the embrace of rational thought. We can blame the intellectual legacy of the Western Enlightenment for this. This inclination leads us to believe that we can attain a specific threshold of certainty and predictability—a notion that, upon closer scrutiny, reveals itself to be an illusory construct detached from our daily experiences and pragmatic realities. We have actually never been rational (a nod to Bruno Latour).
So does having some rudimentary understanding of statistics might help navigate this treacherous world full of probabilistic potholes? We know the difference between mean, median, and mode (do we?). Right? This should help.
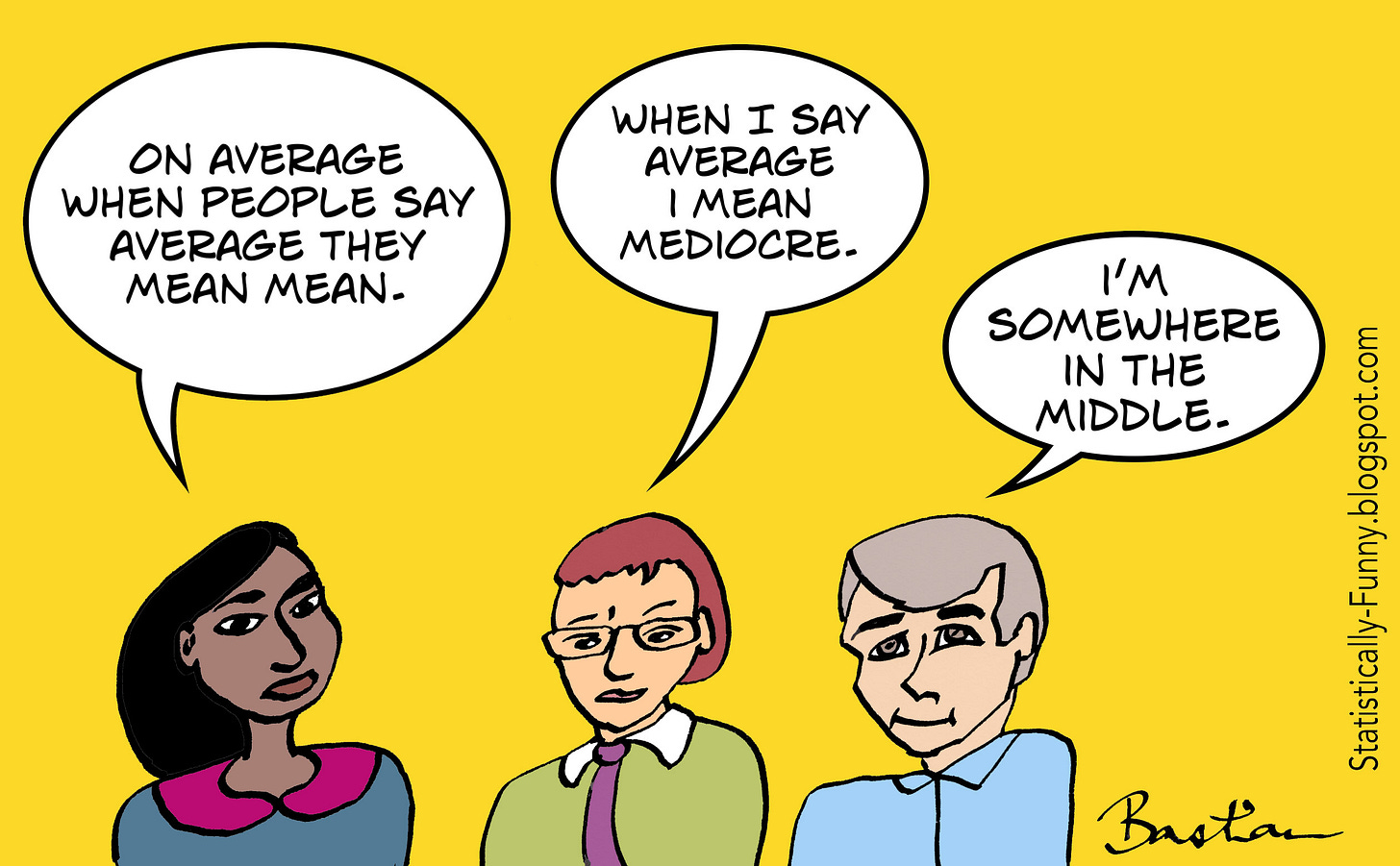
So does statistics stand as a beacon of hope and understanding or just another tool for us to fumble our way through the universe?
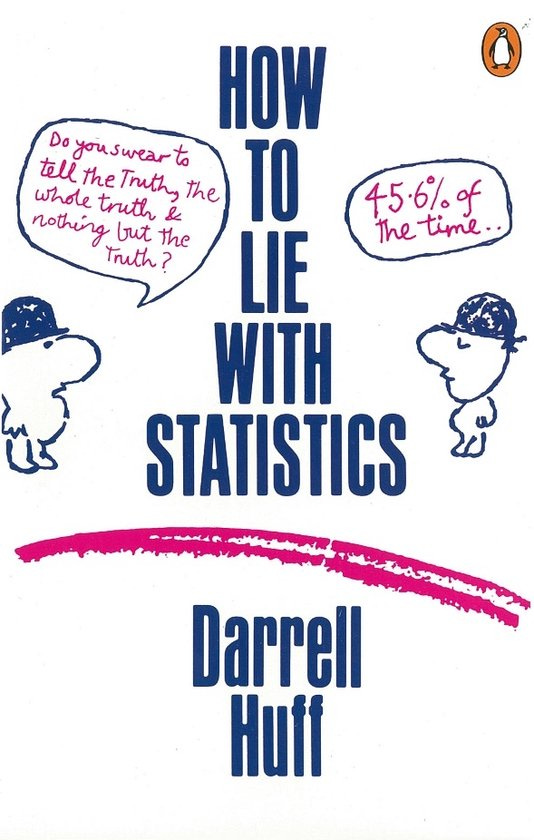
Let’s start with this rain thing.
In 2008, a survey done by the U.S National Center for Atmospheric Research (NCAR) to examine people’s understanding of weather forecasts. One question posed in the survey focused on interpreting a 20 percent chance of rain, offering multiple choice answers. In 2014, NPR reported on this topic and conducted its own survey, featuring an interview with Jordan Ellenberg, the author of How Not To be wrong. Ellenberg explained that the forecast of a 20 percent chance of rain could be interpreted in different ways. One approach involves analysing a database of similar weather conditions and finding that out of 1000 days, rain occurred the following day on 200 occasions, leading to a 20 percent chance of rain. Another interpretation could suggest that it will rain for 20 percent of the time or cover 20 percent of the region.
If we ask a meteorologist, perhaps we can get a better answer:
From meterologist Eli Jacks, of the National Oceanic and Atmospheric Administration's National Weather Service:
"There's a 20 percent chance that at least one-hundreth of an inch of rain — and we call that measurable amounts of rain — will fall at any specific point in a forecast area."
Or ….
.. from Jason Samenow, chief meteorologist with The Washington Post's Capital Weather Gang:
"It simply means for any locations for which the 20 percent chance of rain applies, measurable rain (more than a trace) would be expected to fall in two of every 10 weather situations like it."
hmm..ok, pretty clear? right?
For further insights into how meteorologists make decisions, I recommend exploring "Masters of Uncertainty: Weather Forecasters and the Quest for Ground Truth" (2015) authored by Phaedra Daipha, a cultural sociologist. In her book, Daipha contends that weather prediction will forever remain more of an art than a science. She highlights that meteorologists possess exceptional skills in navigating uncertainty. Notably, they consistently demonstrate superior calibration of their performance compared to professionals in fields such as stockbroking and healthcare. This is primarily due to meteorologists receiving prompt feedback on their decisions, enabling them to continually refine their forecasting abilities.
The NCAR study concluded that “effectively communicating forecast uncertainty to non-meteorologists remains challenging”. Even though weather forecasting technologies got better our understanding of probability or statistics has not improved. In simple terms, determinism implies that if A occurs, then B will undoubtedly follow. However, this line of thinking fails to consider the intricate contextual factors that can influence both A and B. Without a comprehensive understanding of these hidden variables, deterministic thinking merely assumes that when a specific condition arises, the outcome will be certain. For example, we may assert that if a rain forecast predicts heavy rainfall, flooding will occur. Simultaneously, we can argue that if a rain forecast predicts heavy rainfall, it will assist in replenishing the city's water reservoir. In reality, both scenarios can unfold. Thus, we find ourselves once again residing in the realm of uncertainty like Schrödinger's cat. (To be serious for a moment — Schrödinger's Cat experiment would unfold in the following manner: the moment the radioactive atom interacts with the Geiger counter, it collapses from its superposition of non-decayed/decayed states into a definite state. Consequently, the Geiger counter is triggered resulting in the definite demise of the cat. Or, if the Geiger counter is not triggered, the cat is definitively alive. However, both outcomes cannot occur simultaneously).
The trick here is to get out of the binary framework of the deterministic and non-deterministic framework. A lack of certainty does not mean anything can happen. It is simply how we handle the uncertainty with enough data and context and make the decision.
We are not good at statistics and probabilistic thinking because it is hard to embrace uncertainty. However, at the same time so called rationalist thought reminds us that the world should be predictable. These notions have been reinforced by the necessity of government bureaucracy. During the Enlightenment era, as nations aimed to foster social and economic progress, the emergence of national measurement systems became crucial. Statistics became a valuable tool for measuring various aspects. France played a significant role in this development, with prominent French mathematicians like Blaise Pascal and Pierre de Fermat recognising the significance of variability as a serious mathematical problem. In 1833, France established one of the first official statistical bureaus, further elevating the importance of statistics in official decision-making processes. Pascal for example delved into the realm of probabilistic not to calculate the GDP of a nation. He wanted to provide a rationalistic theory of theistic belief. Here’s a famous passage from his work, now widely known as Pascal’s Wager:
“God is, or He is not.” But to which side shall we incline? Reason can decide nothing here. There is an infinite chaos which separated us. A game is being played at the extremity of this infinite distance where heads or tails will turn up… Which will you choose then? Let us see. Since you must choose, let us see which interests you least. You have two things to lose, the true and the good; and two things to stake, your reason and your will, your knowledge and your happiness; and your nature has two things to shun, error and misery. Your reason is no more shocked in choosing one rather than the other, since you must of necessity choose… But your happiness? Let us weigh the gain and the loss in wagering that God is… If you gain, you gain all; if you lose, you lose nothing. Wager, then, without hesitation that He is.
Similar to Pascal, presbyterian reverend Thomas Bayes (Bayes' theorem and Bayesian statistics are named after him). One of the important aspects of Bayesian thinking that the idea that probabilities represent a degree of belief in an event occurring. Non-Bayesian (or frequentist approach) is the idea that the probability of an event occurring is equal to the long frequency with which that event occurs. Bayes’s idea of such belief came because of his argument with David Hume about God and miracle such as Christ’s resurrection:
It all began in 1748, when the philosopher David Hume published An Enquiry Concerning Human Understanding, calling into question, among other things, the existence of miracles. According to Hume, the probability of people inaccurately claiming that they’d seen Jesus’ resurrection far outweighed the probability that the event had occurred in the first place. This did not sit well with the reverend.
Inspired to prove Hume wrong, Bayes tried to quantify the probability of an event. He came up with a simple fictional scenario to start: Consider a ball thrown onto a flat table behind your back. You can make a guess as to where it landed, but there’s no way to know for certain how accurate you were, at least not without looking. Then, he says, have a colleague throw another ball onto the table and tell you whether it landed to the right or left of the first ball. If it landed to the right, for example, the first ball is more likely to be on the left side of the table (such an assumption leaves more space to the ball’s right for the second ball to land). With each new ball your colleague throws, you can update your guess to better model the location of the original ball. In a similar fashion, Bayes thought, the various testimonials to Christ’s resurrection suggested the event couldn’t be discounted the way Hume asserted.
So does this mean that Bayesian understanding is problematic because of such theistic past? Not really. It is indeed interesting to acknowledge the historical roots of our ideas. Moreover, belief systems and subjectivity persist even within what we perceive as scientific rigour. One prominent example is the widely used statistical threshold known as the p-value, considered the gold standard for assessing statistical significance. This concept was introduced by Ronald Fisher, a devout Anglican and notable British geneticist and statistician, back in 1925. Fisher proposed the p-value as a measure of the significance of data in relation to a proposed model. Remarkably, the value of 0.05 has become a cornerstone in thousands upon thousands of studies. However, it is essential to recognise that Fisher arrived at this specific number arbitrarily and for convenience. It serves as a simple threshold that is easily comprehensible, rather than dealing with more complex values like 0.93 or 0.71.
This does not mean we should abandon statistics. We just need to embrace uncertainty and find better ways to identify the odds. We can never know the future with exact precision. But properly understanding and applying statistics with all the limitations can be a big asset. Take rain forecast or even cancer diagnostic, a 100% chance of a positive test does not necessarily mean a dire diagnosis. Learning from Bayesian history reminds us that the resurrection of Christ was a low prior probability event, yet it's still the foundation of an entire belief system. So, as we dance between the realms of randomness and reason, let us embrace the power of probabilistic thinking. It may not always provide definite answers, but it opens the door to a world where curiosity and uncertainty coexist in a waltz of enlightenment.
This has me thinking of a letter that the poet John Keats wrote to his brothers that includes this passage: "several things dovetailed in my mind, & at once it struck me, what quality went to form a Man of Achievement especially in Literature & which Shakespeare possessed so enormously – I mean Negative Capability, that is when a man is capable of being in uncertainties, Mysteries, doubts, without any irritable reaching after fact & reason – Coleridge, for instance, would let go by a fine isolated verisimilitude caught from the Penetralium of mystery, from being incapable of remaining content with half knowledge."