Predictive Government: From 'Minority Report' to 'Majority Worries'
Exploring AI in Government: From Allegheny County to the Wider World
Dear Readers,
Have you ever visited Allegheny County in Southwestern Pennsylvania? It is beautiful, especially this time of year, with falling leaves and autumn weather all around. But we are here to talk about AI. Read on to see why we’re mentioning Allegheny County. And do you remember the movie Minority Report (2002)?
Governments worldwide are increasingly utilising AI and automated decision-making systems that impact various aspects of people’s lives. While AI has the potential to enhance efficiency and allow humans to focus on areas where their unique skills shine, unchecked use of these technologies in government applications is a growing concern. This issue is not limited to the United States but extends to many countries globally.
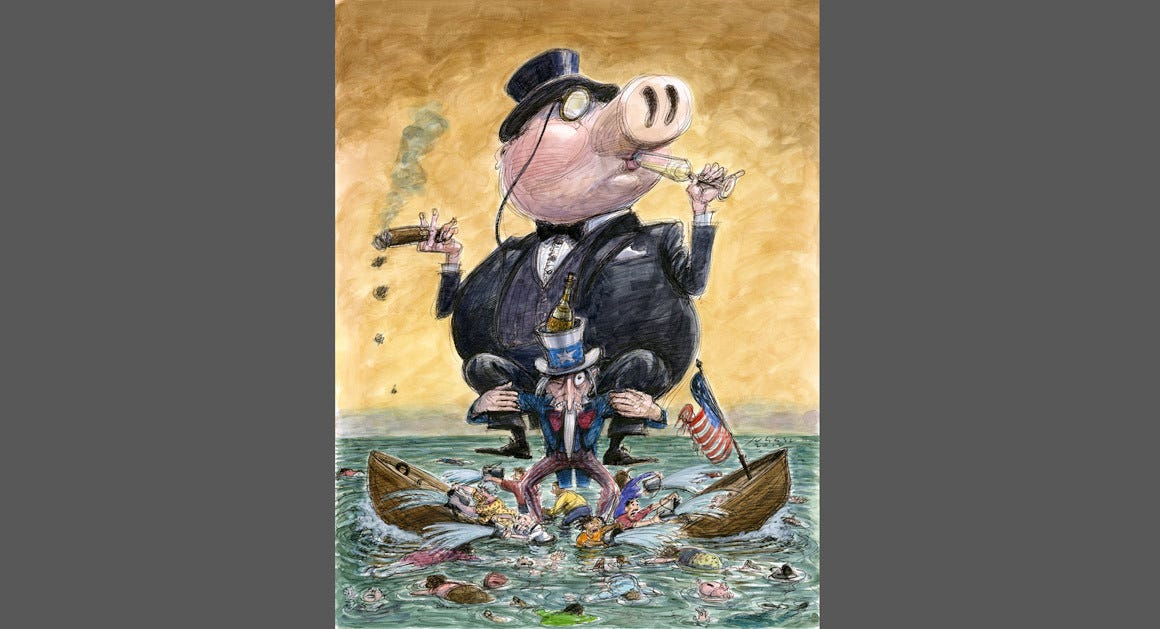
In the U.S., AI already found its way into the criminal justice system, where its deployment tends to exacerbate existing discriminatory patterns. For instance, studies have shown that facial recognition tools are significantly more likely to produce false positives for non-white individuals and darker-skinned females (such as false accusations ad arrests). Similar challenges are observed in Europe even though privacy and data protections law are stricter there than in the U.S:
Across Europe, police, migration and security authorities are seeking to develop and use AI in increasing contexts. From the planned use of AI-based video surveillance at the 2024 Paris Olympics, to the millions of EU funds invested in AI based surveillance at Europe’s borders, AI systems are more and more part of the state surveillance infrastructure.
We’re also seeing AI deployed with the specific purpose of targeting specific communities. Technologies like predictive policing, whilst presented as neutral tools in the fight against crime, have as their basis the assumption that certain groups – in particular racialised, migrant and working class people, are more likely to commit crime.
In the Netherlands, we have seen the vast consequences predictive policing systems have for Black and Brown young people. The Top-600, a system designed for the ‘preventive identification’ of ‘potential’ violent criminals, was found, following investigation, to disproportionately over-represent Moroccan and Surinamese suspects.
Media often extensively covers the latest features of chatGPT, yet there is a notable lack of attention to policy issues. One pressing concern revolves around the use of AI in public benefit administration, specifically in the tracking and assignment of “risk scores” to recipients of these benefits. These scores can be influenced by seemingly inconsequential factors, resulting in unjust repercussions. Several countries, including France, the Netherlands, Denmark, Ireland, Spain, Poland, and Italy, have implemented AI to combat welfare fraud. However, these endeavours have sparked controversy and errors, exemplified by the Dutch childcare benefits scandal. It’s important to note that some of these reactions regarding scrutiny around welfare fraud are politicised, often in response to the arrival of recent migrants and refugees (as some European governments are spending social welfare resources for the refugees and asylum seekers also implementing measures to restrict immigration). From The Wired, March 7, 2023.
Denmark isn’t alone in turning to algorithms amid political pressure to crack down on welfare fraud. France adopted the technology in 2010, the Netherlands in 2013, Ireland in 2016, Spain in 2018, Poland in 2021, and Italy in 2022. But it’s the Netherlands that has provided the clearest warning against technological overreach. In 2021, a childcare benefits scandal, in which 20,000 families were wrongly accused of fraud, led to the resignation of the entire Dutch government. It came after officials interpreted small errors, such as a missing signature, as evidence of fraud, and forced welfare recipients to pay back thousands of euros they’d received as benefits payments.As details of the Dutch scandal emerged, it was found that an algorithm had selected thousands of parents—nearly 70 percent of whom were first or second generation migrants—for investigation. The system was abandoned after the Dutch Data Protection Authority found that it had illegally used nationality as a variable, which Amnesty International later compared to “digital ethnic profiling.”
Decision about public housing allocations are also impacted by AI, influencing lending decisions and tenant screening. The Electronic Privacy Information Center (EPIC), a U.S.-based non-profit organisation, has shed light on the widespread use of AI systems in state and local governments. These systems, operated by private companies like Deloitte, Thomson Reuters, and LexisNexis, make significant government decisions without public input or oversight. EPIC maintains a database of companies receiving U.S. government contracts, highlighting the intricate network of organisations involved in AI adoption.
Another great source of such information in Opensecrets:“Nonpartisan, independent and nonprofit, OpenSecrets is the nation's premier research group tracking money in U.S. politics and its effect on elections and public policy. Our mission is to track the flow of money in American politics and provide the data and analysis to strengthen democracy.”
From there and also via
I learned about Dataminr, a big player but relatively lesser-known company that has played a significant role in social media surveillance for government agencies.The bulk of Dataminr's U.S. federal income has resulted from its (ongoing) $267 million contract with the U.S. Air Force (there the legal term of art for social media surveillance is "publicly available information" monitoring). Dataminr's newly signed contract with the Drug Enforcement Administration -- whose Office of National Security Intelligence is one of eighteen members of the U.S. Intelligence Community -- is at odds with the widespread reporting in 2016 that Twitter "cut off" intelligence agencies from its platform. (Telegram has since become an increasingly central target of government surveillance, with firms such as Flashpoint and Nisos openly selling access to the information they gain from infiltrating private Telegram groups.)
Check out Jack’s Substack:
Now, let’s go to Pennsylvania.
One under-the-radar example of AI’s impact is the Allegheny Family Screening Tool (AFST), a seemingly run of the mill government tool. But it uses predictive techniques to assess the risk of child removal from homes for safety concerns. While it has been in use since 2019, the tool recently has faced criticism and U.S DOJ investigation for potential bias against low-income and minority families. It was developed by a team of experts led by Professor Rhema Vaithianathan of the University of Auckland, New Zealand.
In 2022, a study published by ACLU found that the AFST was more likely to predict that Black children and children from low-income families would be removed from their homes, even when they were not at any greater risk than white children or children from high-income families. This prediction often gets passed on to different decision-making chains within the welfare system without proper questioning and scrutiny. Importantly, many decision-makers down the line have no clue about how the prediction was generated. Even though the authors of this tool emphasised that AFST should not be used as the sole means, a detailed examination of the process and design of the algorithm reveals potential issues that remain unchecked within current government structures. It’s worth noting that even when humans are in the loop, there are challenges in understanding of how AI prediction fits into the rest of the structure. The policy and governance aspects are likely to change in the near future; therefore, the media and the public need to seek sources of information to comprehend these AI related issues. Studies conducted by EPIC and the ACLU are crucial for gaining such understanding. It’s important to emphasise that this is not about criticism for criticism's sake or raising objections just for the fun of it. I fully support the proper use of AI, with transparency, provenance, and oversight. However, we must delve into the design considerations that impact a significant number of people. Here’s an example from AFST, involving “risk by association.” Even though humans are involved in this process, the design biases and policy enforcement lack transparency. As highlighted in the ACLU report:
In creating the AFST, the developers of the tool made several consequential decisions about how to present risk scores to screening staff, ultimately transforming the model’s outputs — predicted probabilities for individual children — into the format shown to call screeners — a single risk label or numeric score between 1 and 20 representing all children on a referral. In this section, we analyze this series of post-processing decisions [87] related to the aggregation and communication of the AFST’s outputs. We argue first that these decisions are effectively policy choices, and that the AFST’s method of grouping risk scores presents a misleading picture of families evaluated by the tool, treating families as “risky” by association, even when the risk scores of individual family members may be perceived as low. Viewing these decisions as policy choices, we highlight several additional ways these decisions could have been analyzed throughout the AFST’s design and deployment process, which produce varying pictures of how the tool performs.
The AFST aggregates risk scores for all children in a household, presenting a single score or label that represents an entire family. This can be misleading, as it treats families as “risky” by association, even when the risk scores of individual family members may be perceived as low.
The choice of whether to include or exclude variables from the juvenile probation system in the AFST is a policy choice, and not solely a technical decision. Even if accuracy is the primary consideration, model multiplicity could allow tool developers to prioritize other considerations, such as fairness and interpretability.
Furthermore, the tool aggregates the scores of all children within a household into a single risk label or score, which can create confusion about which child’s risk is under consideration. It’s important to bear in mind that the predictive decision is relayed across various levels within the agency. This complexity is amplified by AI, as different officials are conveying information, interpretations, and understanding at different stages — from algorithm designers and decision-makers in the county to welfare system officials.
Imagine a referral related to a hypothetical family with three children, aged 5, 10, and 15 respectively, with AFST scores of 5, 10, and 18. One child, the 5-year-old child with a risk score of 5, is labelled as the alleged victim by the county on the referral. How could this information be communicated to the call screener for the referral? As noted in Section 3, the county has a policy of evaluating all of the children on a referral when a call is received — not just those indicated as alleged victims — and this policy pre-dates the AFST [91, p. 14]. But the existence of this policy alone does not answer this question of how scores are communicated to call screeners. For example, one option would be to show each child’s individual score to the call screener, for a total of three scores. Or, with a constraint of only showing one score, the AFST could have displayed the score of the alleged victim (a score of 5), or the maximum score of all children (a score of 18), or a label such as “high-risk” for the entire household based on the score and the children’s ages, akin to the county’s current protocol policy. Under the policy that, to our knowledge, is currently in use in the county, this family would be grouped into the “high-risk protocol.”
Again, this is not to suggest we should ban the use of such tools. The key issue is that predictive risk models like AFST are not impartial, and their use raises concerns, especially when they incorporate arbitrary choices, lack opportunities for families to challenge the results, perpetuate racial biases, and unfairly label people. While there is significant discussion about ethical and safe AI, there’s relatively little effort to truly “understand” how algorithms can promote justice by considering their broader social and economic contexts. The challenge lies in bridging our human notion of “understanding” with the generative and predictive speed and ease of AI. It’s important to remember that while it’s nice to “predict” the next sequence of texts and generate images rapidly, it becomes an entirely different challenge when those predictions are tied to policy structures and affect economically vulnerable populations.
I will leave you with the following excerpt from the book In AI We Trust, by Helga Nowotny, Professor emeritus of Social Studies of Science, ETH Zurich:
The power of algorithms to churn out practical and measurable predictions that are useful in our daily lives — whether in the management of health systems, in automated financial trading, in making businesses more profitable or expanding the creative industries — is so great that we easily sidestep or even forget the importance of the link between understanding and prediction. But we must not yield to the convenience of efficiency and abandon the desire to understand, nor the curiosity and persistent that underpin it (Zurn and Shankar 2020).
…..
Understanding also includes the expectations that we can learn how things work. If an AI system claims to solve problems at least as well as a human, then there is no reason not to expect and demand transparency and accountability from it. In practice, we are far from receiving satisfactory answers as to how the inner representations of AI work in sufficient detail, let alone an answer to the question of cause and effect. The awareness begin to sink in that we are about to lose something connected to what makes us human, as difficult to pin down as it is.
…
After all, what makes us human is our unique ability to ask the question: Why do things happen - why and how?
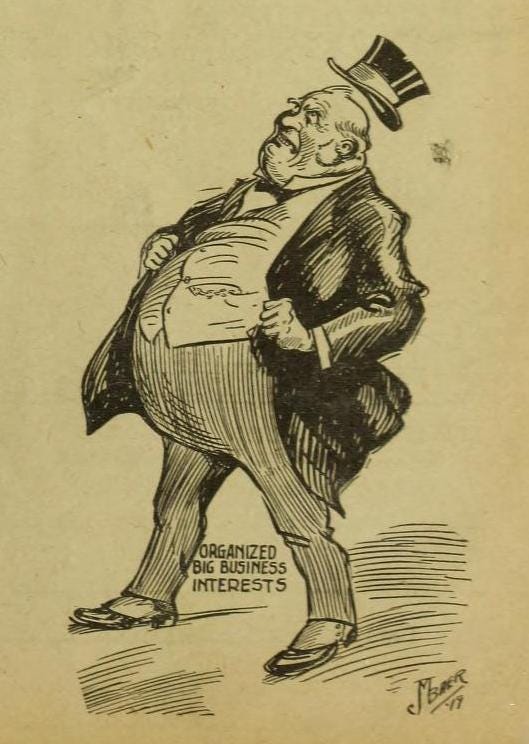
And a “Fat Cat” caricature from 1919.