A few weeks ago, I attended a workshop where we encountered a “manel” — a panel comprising solely male experts or participants. This issue isn’t new; discussions on rectifying such situation is also not. However, remedies often resort to token representation or generic gender and ethics workshops, lacking genuine constructive efforts. Addressing this problem isn’t straightforward, even with good intentions, due to structural barriers and insufficient expertise. Moreover, awareness plays a significant role; initiatives like declining participation in all-male panels or expanding professional networks are steps in the right direction. However, what often gets overlooked in these token fixes is the crucial aspect of context — understanding why diversity and pluralistic perspectives are vital and how it can help. Also to acknowledge the fact that diversity encompasses multiple dimensions beyond gender, and engaging in nuanced contextual discussions proves challenging.
This issue of context intersects with AI in various ways. While AI can conduct amazing sentence-level context analysis, its comprehension of specific needs and nuances remains limited. The recent controversy surrounding Gemini exemplifies this.
Gemini’s attempt at inclusivity ended up exacerbating the problem, akin to adding token representation without addressing the root issues. Despite considerable investment in AI technology, models like Gemini still struggle with basic details and lack true understanding. They operate based on pre-existing data rather than genuine knowledge or intelligence.
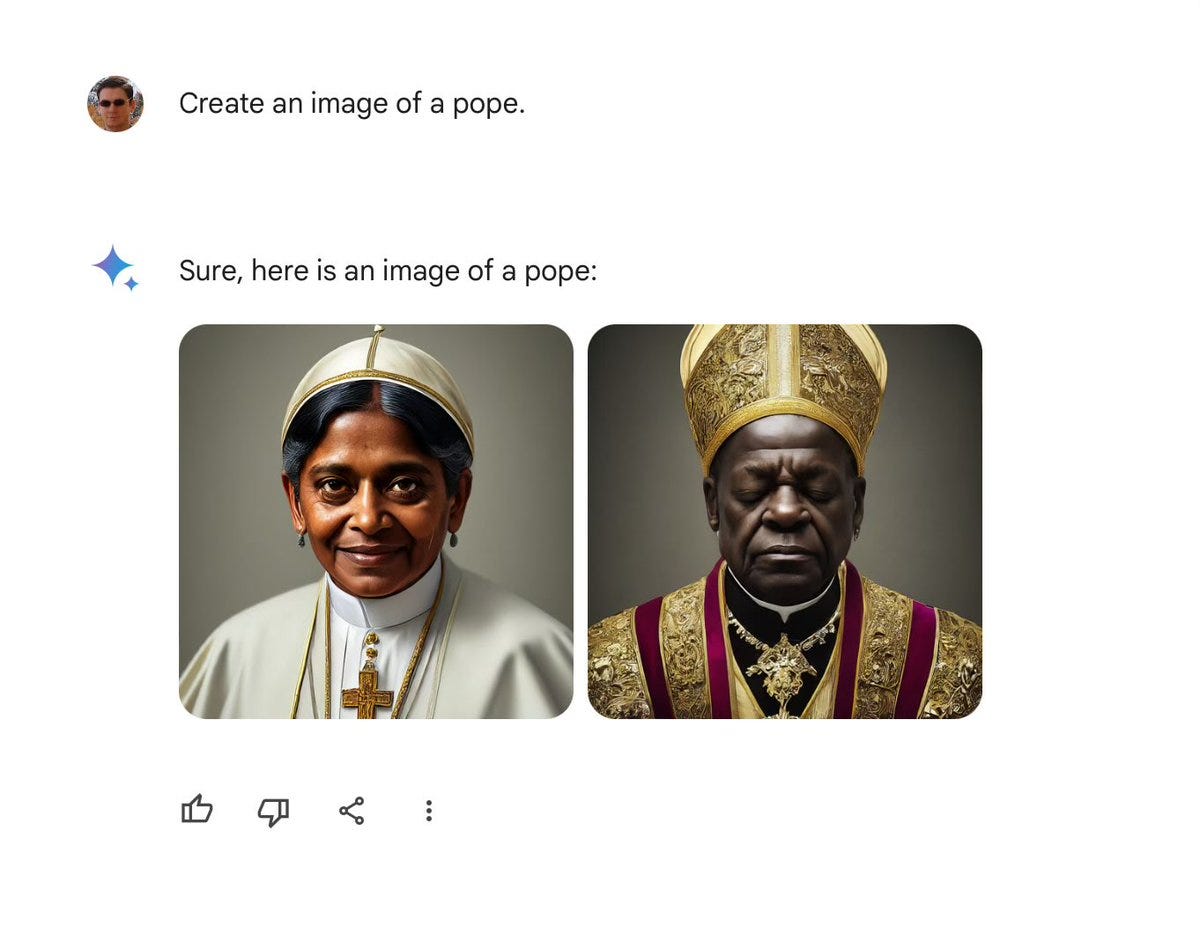
Sora's outputs can mimic real-life objects in a genuinely chilling way, but its outputs — like DALL-E, like ChatGPT — are marred by the fact that these models do not actually know anything. They do not know how many arms a monkey has, as these models do not "know" anything. Sora generates responses based on the data that it has been trained upon, which results in content that is reality-adjacent, but not actually realistic. This is why, despite shoveling billions of dollars and likely petabytes of data into their models, generative AI models still fail to get the basic details of images right, like fingers or eyes, or tools.
These models are not saying "I shall now draw a monkey," they are saying "I have been asked for something called a monkey, I will now draw on my dataset to generate what is most likely a monkey." These things are not "learning," or "understanding," or even "intelligent" — they're giant math machines that, while impressive at first, can never assail the limits of a technology that doesn't actually know anything.
This is not to undermine the technological progress. No doubt, the recent advancement in AI is amazing. But we often forgot it's difficult to design and manage LLMs. They’re not very dependable and are better suited for specific tasks. However, when used on a wide scale even minor issues can cause major public relations problems.
From
Gemini sparked political rage this week by generating images of historical people and groups with unexpected ethnic diversity. The eyebrow-raising performance likely results from Google coaching the models to respond with diverse types of people, addressing the well-identified “CEO vs receptionist” problem of its search results. Google aimed to reduce bias in its models (which stems from biases within its internet training data), but the outcome compromised historical accuracy.
Our experience of the world is going to be filtered by AI systems. The people producing them need to act with a greater degree of transparency.
And also another round of the market hype rollercoaster, brought to you by our friendly neighbourhood snake-oil salesman, NVIDIA CEO. Apparently, even those who can barely distinguish a keyboard from a frying pan are spewing out more BS than a fertiliser factory. Can you imagine the deluge when they officially stop teaching programming? Maybe we’ll switch to Comparative Theology 101 for the kiddos? Oh, the joys of AI!
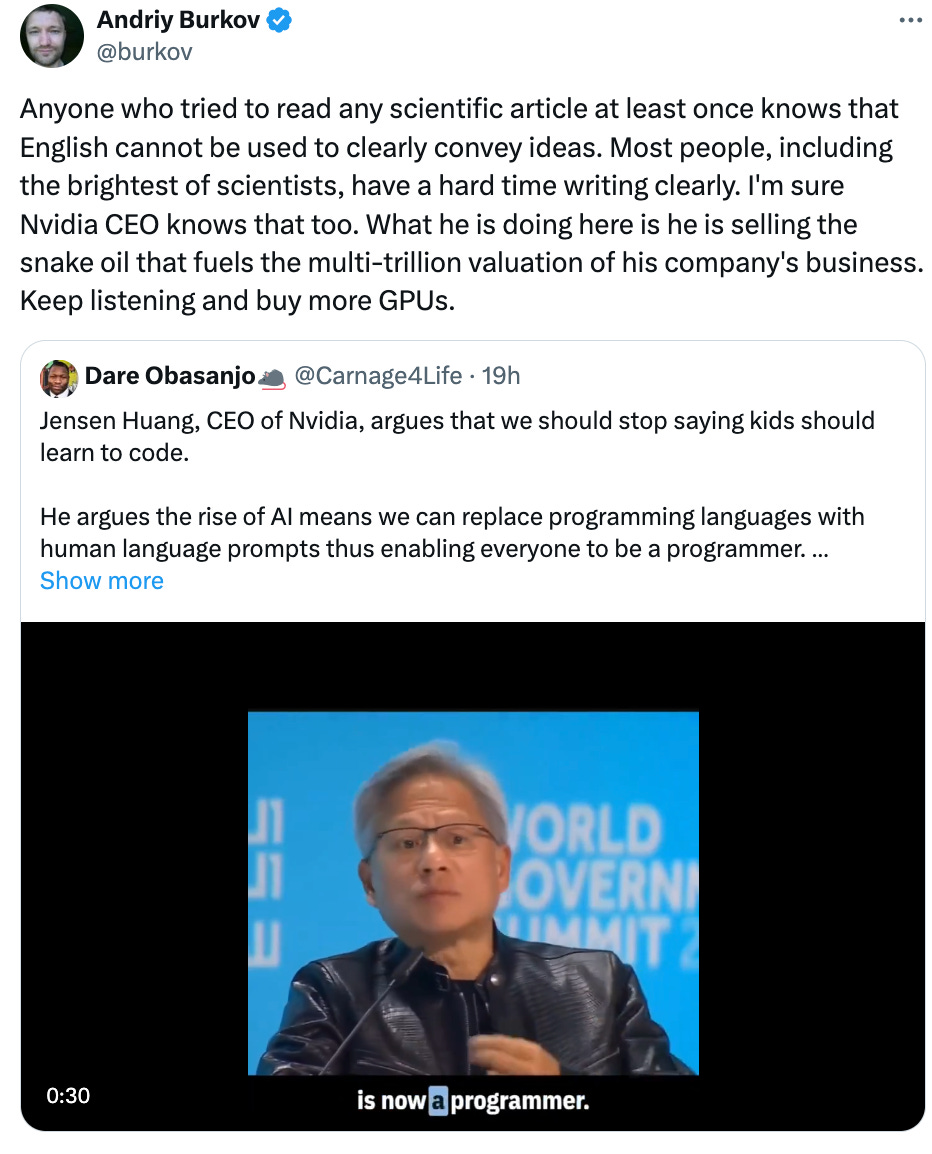
Yes, there’s also something known as a large context window and token (not the token representation I mentioned earlier, apologies for the pun). Gemini 1.5 now operates with a 1-million-token context window. However, this didn’t prevent the subsequent woke controversy. It appears that scale and size weren’t relevant factors here.
And spare me the notion that RAG will solve everything. RAG (Retrieval Augmented Generation) in AI enhances Generative AI applications by providing relevant contextual data during tasks. Yet, this context differs significantly from understanding social and political complexities, such as issues like diversity. We humans fail at it miserably and yet expect our models to excel? From
I do think AI will eventually work reliably. Some great-grandchild of RAG, perhaps extended, revised, and elaborated, might even help — if people seriously embrace neurosymbolic AI in coming years.
But we are kidding ourselves if it we think the solution is near at hand. Making it work is very likely going to require a whole new technology, one that is unlikely to arrive until the tech industry gets over its infatuation with LLMs and quick fixes.
We need to push for more openness and transparency from the AI snake-oil merchants. This was the token apology from a Senior Vice President of Google,
This wasn’t what we intended. We did not want Gemini to refuse to create images of any particular group. And we did not want it to create inaccurate historical — or any other — images. So we turned the image generation of people off and will work to improve it significantly before turning it back on. This process will include extensive testing.
One thing to bear in mind: Gemini is built as a creativity and productivity tool, and it may not always be reliable, especially when it comes to generating images or text about current events, evolving news or hot-button topics. It will make mistakes. As we’ve said from the beginning, hallucinations are a known challenge with all LLMs — there are instances where the AI just gets things wrong. This is something that we’re constantly working on improving.
While they express regret for the feature’s shortcomings, it’s evident that more needs to be done. But given Google’s history with AI ethics, this sort of half hearted effort is not surprising. The Gemini incident underscores the necessity for Google and other’s to prioritise thorough testing and broader discussion. I leave you with this reflection from Yacine Jernite, machine learning and society lead at Hugging Face,
Bias is compounded by choices made at all levels of the development process, with choices earliest having some of the largest impact—for example, choosing what base technology to use, where to get your data, and how much to use. Jernite fears that what we’re seeing could be the result of what companies see as implementing a quick, relatively cheap fix: If their training data overrepresents white people, you can modify prompts under the hood to inject diversity. “But it doesn’t really solve the issue in a meaningful way.
Can we move past the snake-oil sales pitch of AI?
I’m not holding my breath, but hey, who knows what the future holds? But remember, often this not about AI. We’ve got some deep-rooted human issues to tackle first, and there’s no quick technical fix for those.